Overview
At Preferred Networks, Inc. (PFN), we work day in and day out on the research and development of personal robots. Our aim is to create a society where robots can actively support our daily living activities.
At CEATEC JAPAN 2018, PFN will use HSRs (Human Support Robots) developed by Toyota Motor Corporation※ to demonstrate a fully-autonomous tidying-up robot system that leverages cutting-edge deep learning technology.
The robot system has been awarded the Semi-Grand Prix in the Industry/Market category at CEATEC Award 2018, which recognizes innovative technologies, products, and services from among a large number of exhibits at CEATEC JAPAN 2018.
This system is the first of its kind that can automatically keep a cluttered room neat and tidy at a practical level, something that has been difficult to achieve using conventional robot system. Thanks to the rapid advancement of deep learning technology in recent years, PFN has utilized cutting-edge deep learning techniques to enable the robot to recognize objects and understand spoken language as well as controlling the robot. As a result, the robot is able to quickly and accurately grasp and place objects, how to plan its movements, follow human instructions, all of which are essential for a robot to work in the human living space.
※ PFN has participated in the HSR developer community and made use of HSR hardware and software platforms.
Object Detection
To date, robots have been used mainly in factories. In factory lines, robots usually handle limited types of items that are always brought right in front of them enabling them to work fast and precisely.
However, personal robots need to handle a great variety of items in the living space while easily responding to complex and dynamic situations. PFN has developed a computer vision engine based on advanced deep learning technology, which enables the robot to identify the type and location of each object among several hundred types of objects scattered in a room.
Based on the engine, the robot can plan which object to pick up and how to grasp and put it back.
The computer vision engine was made possible by a CNN (Convolutional Neural Network) model that has been trained on the Chainer deep learning framework, ChainerMN and ChainerCV. The CNN is an extended PFDet model that won second place in an international object detection competition held in September. More than 100 GPUs of the large-scale GPU cluster MN-1b were used to train the model.
This movie shows how the robot sees its surroundings from the camera, and how it recognizes objects in its view.
This visualization shows what the room looks like in the robot’s mind. It displays the map of the room recognized by the robot at its current location.
Picking & Placing Objects
There are various kinds of things in the human living space – from things having no definite shape like handkerchiefs, long and thin items like pens, to items like small clips which are difficult to pick up.
This system can stably pick up and place objects of various shapes and materials in their designated locations.
Take a pen for example, the robot searches for a pen holder using its camera. After recognizing and aligning the orientation of the pen, it puts the pen in the holder.
The robot is also capable of stably grasping amorphous objects such as clothes and socks.
These seemingly easy tasks can only be accomplished by making a number of small decisions that humans make unconsciously.
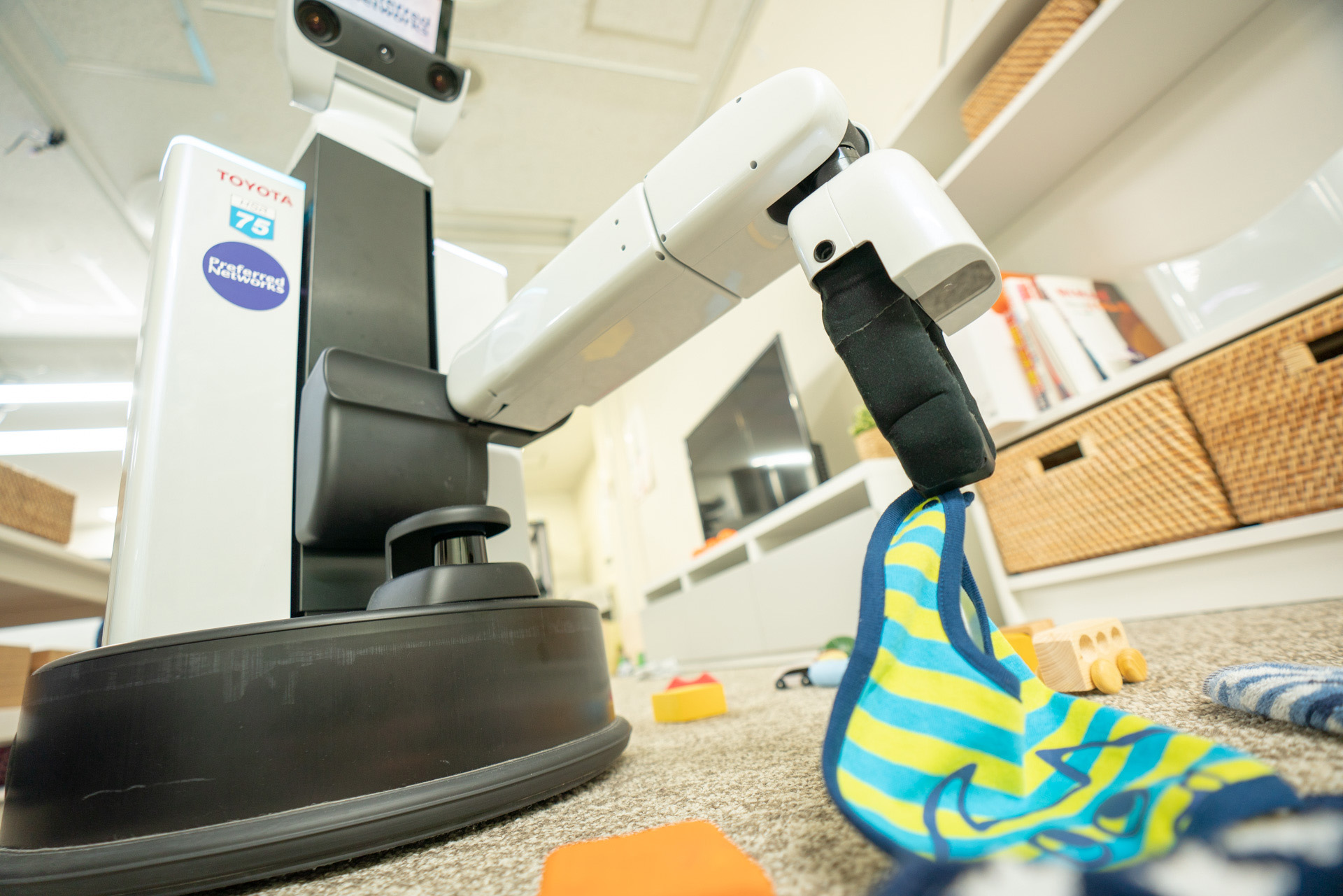
Human Interaction
Conventional industrial robots have been used only by robot professionals who can operate a special control panel. In order to make robots available for everyone, we must allow for more intuitive means of controlling them. PFN’s robot system understands verbal instructions and finger-pointing gestures. With this technology, we can tell the robot to perform a task verbally as if we were talking to a person.
The robot keeps track of everything inside the room and can tell where they are when asked.
In addition, using augmented reality (AR) technology can show what the robot is thinking. You can see a visual display of information on how it recognizes objects in the room and what kind of action it is planning to take next.
By looking at this AR screen, we can intuitively assess the status of the robot. This allows us to give clearer instructions.
Our Team
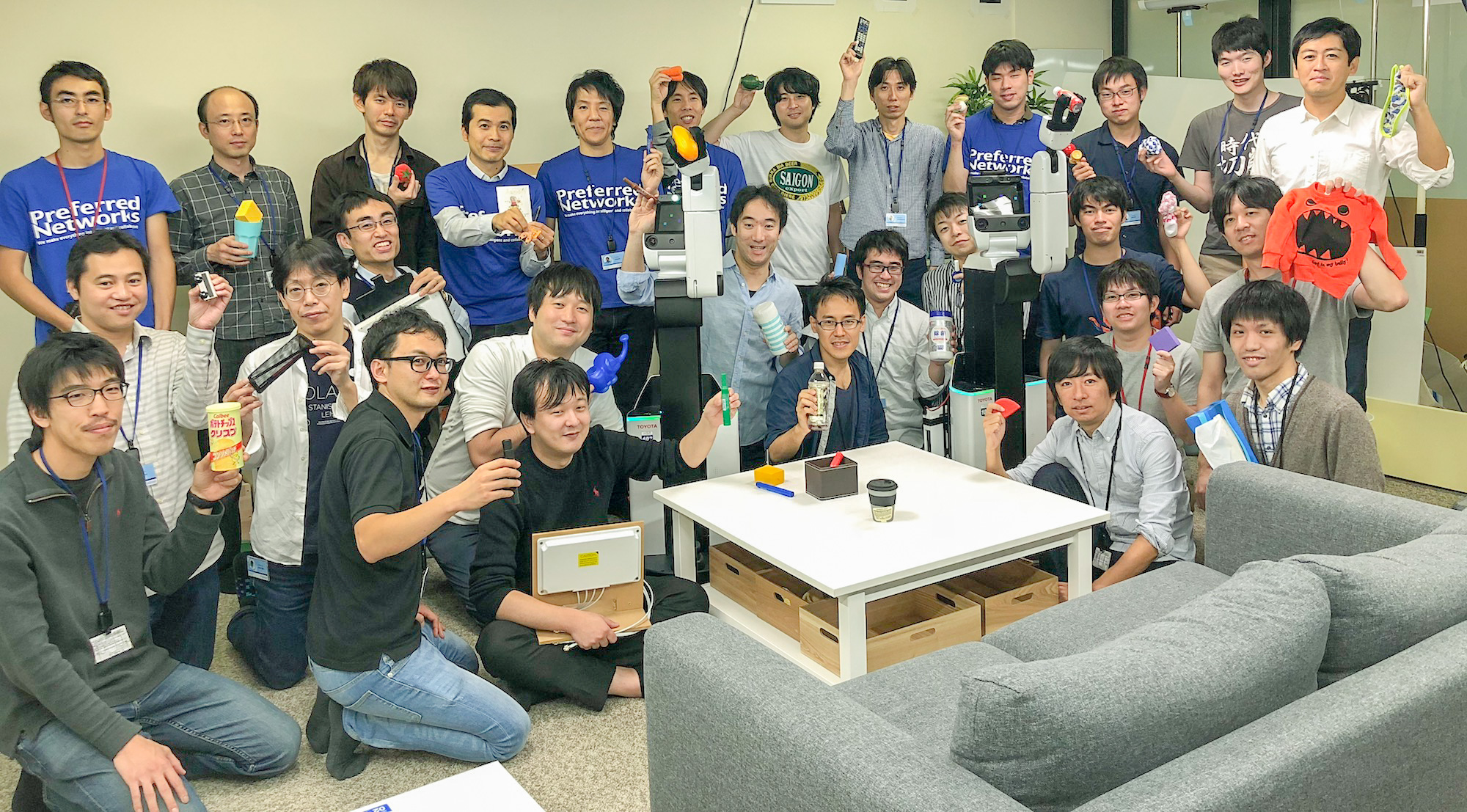
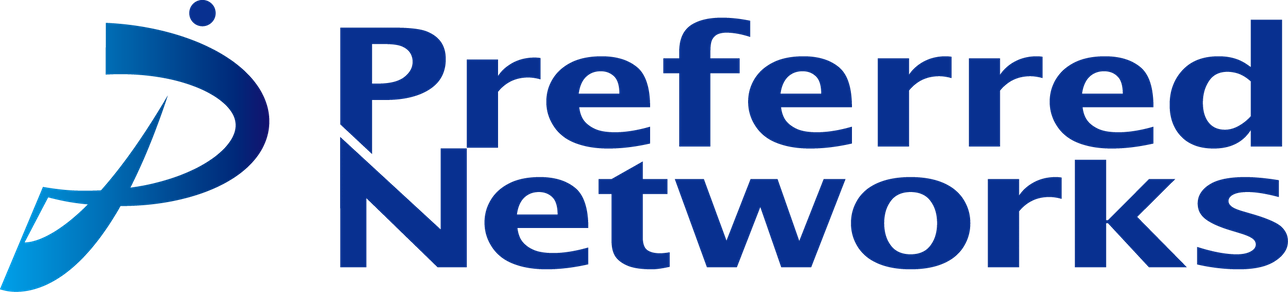
Founded in March 2014 with the aim of promoting business utilization of deep learning technology focused on IoT, PFN advocates Edge Heavy Computing as a way to handle the enormous amounts of data generated by devices in a distributed and collaborative manner at the edge of the network, driving innovation in three priority business areas: transportation, manufacturing and bio/healthcare. PFN develops and provides ChainerTM, an open source deep learning framework. PFN promotes advanced initiatives by collaborating with world leading organizations, such as Toyota Motor Corporation, Fanuc Corporation and the National Cancer Center of Japan.
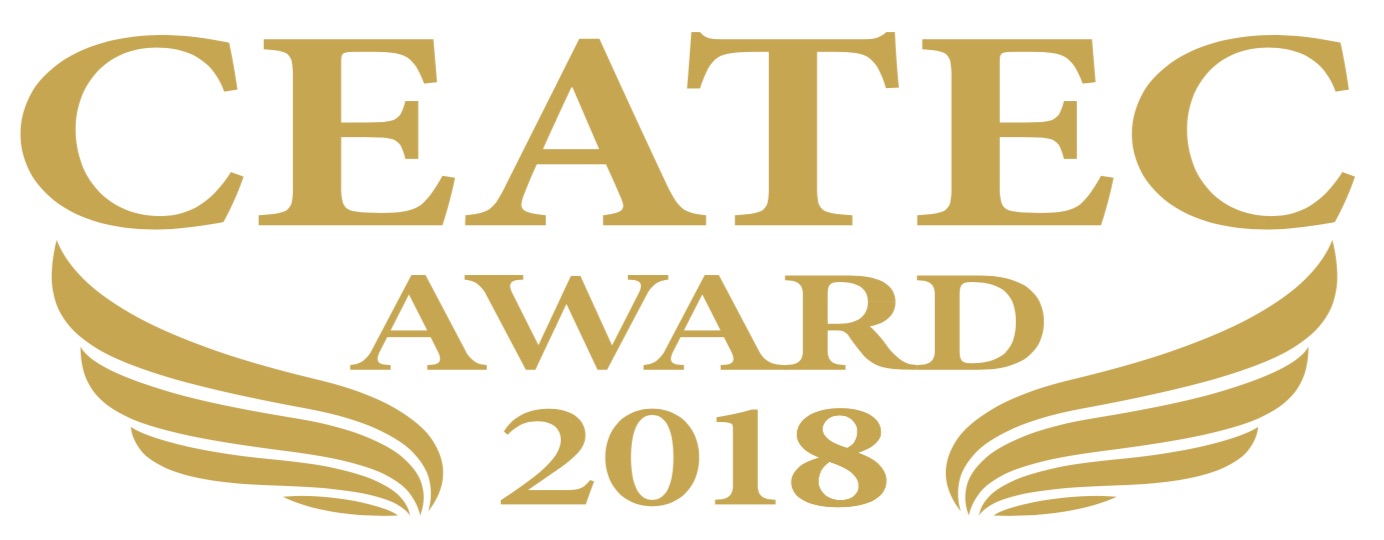
The robot system has been awarded the Semi-Grand Prix in the Industry/Market category at CEATEC Award 2018, which recognizes innovative technologies, products, and services from among a large number of exhibits at CEATEC JAPAN 2018.
The presented system can handle the task of tidying up a room to a practical level of accuracy, which has been difficult to achieve using conventional systems. If the system is commercialized with higher added value, it will have a major impact on the market and society. As well as being a promising product in the area of big data, it has the potential to create new business opportunity as a data library provider for service robots.
Media Coverage
2018/10/15 Wall Street Journal Robot Solves a Problem Kids Can’t: Cleaning Their Rooms
2018/10/16 KYODO NEWSJapanese AI venture develops autonomous tidying-up robot
2018/10/16 Ruptly “Time for a new boyfriend? Super-clean robot will tidy up your home!”
2018/10/16 Bright Deze robots ruimen je rommel op / これらのロボットはあなたの部屋を片付ける
2018/10/17 LCI “Première mondiale au CEATEC de Tokyo : plus fort que le robot aspirateur, voici le robot qui range” [Video]
2018/10/18 Eenadu (India) Watch: Dancing and tidying robots steal the show at Ceatec /
2018/10/19 Le Soir (Belgium) Un robot qui fait le ménage à votre place
2018/10/21 Almasdar Tech فيديو| روبوت ياباني يمكنه ترتيب غرفة طفلك وإعادة كل شيء إلى مكانه
2018/10/22 Robotics Research Personal robot to tidy your room
2018/10/16 Cool3c.com CEATEC 2018 :家裡客廳被亂丟的玩具弄得一團亂? Preferred Networks 結合 Toyota 機器人幫你把散落一地的物品歸位